
We make sure that every organization in the underlying datasets our client has matches the data that’s coming from Thomson Reuters. The final step is stitching, joining and linking the data together. Within our data fusion platform, you can connect and join these different datasets together. The transform step is really important as well, because you have to think about the property graph nodes and relationships you want to create, and how you want to model the data around those relationships. We extract our knowledge graph from our internal graph storage system, which includes meaning, and we deliver it.
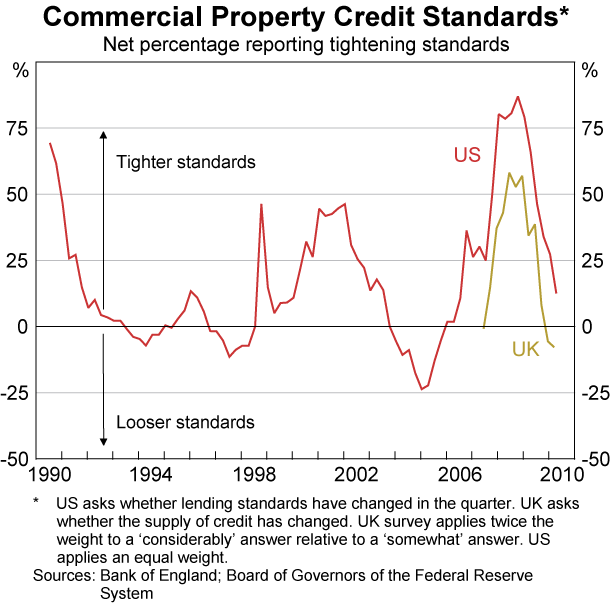
It provides the foundation, but next you need a tool to deliver all the information. The knowledge graph is the core asset we deliver, but that’s just the beginning. It’s pretty big, and you can do a lot with it. It represents about two billion RDF triples and 130 billion paths.
#Thomson reuters graph builder professional#
This is the data we deliver every day to our professional customers through our knowledge graph. 200 million strategic relationships with information that answers the questions: who are my competitors? Who are my suppliers? Who has a joint venture to build this new product? Who’s an ally? Which industry am I in? What is the family tree of this organisation?.75 million metadata objects, such as countries, regions, and cities, that add meaning and context to our data.125 million equity instruments listed on any market anywhere in the world, organized in a highly normalised and described way.This data goes back to the mid-1980s and includes the officers and directors of every single public company, along with their entire work history and the companies they’ve worked for. Management may say one thing, but the reality might be very different – which you can understand from our graph.īelow are the data types included in our knowledge graph: They are the strategic relationships around a company that might help you understand what it’s actually doing. This is represented on the left-hand side of the slide below: We’ve been mining and using natural language processing (NLP) on news and on structured text for over 15 years. We provide highly-structured, highly-normalised content analysts, human expertise, a huge partner network and an understanding of text and structured data. We have 50,000 employees in 100 countries who spend every day gathering and databasing millions of bits of data. Let’s dive into the components of our graph stack. And the third part of our stack requires us to think about the financial analyst so we can come up with a way to deliver a tool that’s genuinely useful. The second part of our stack allows us to carefully link, stitch and join our data together in order to provide useful financial data insights, which we can accomplish through a graph ETL.
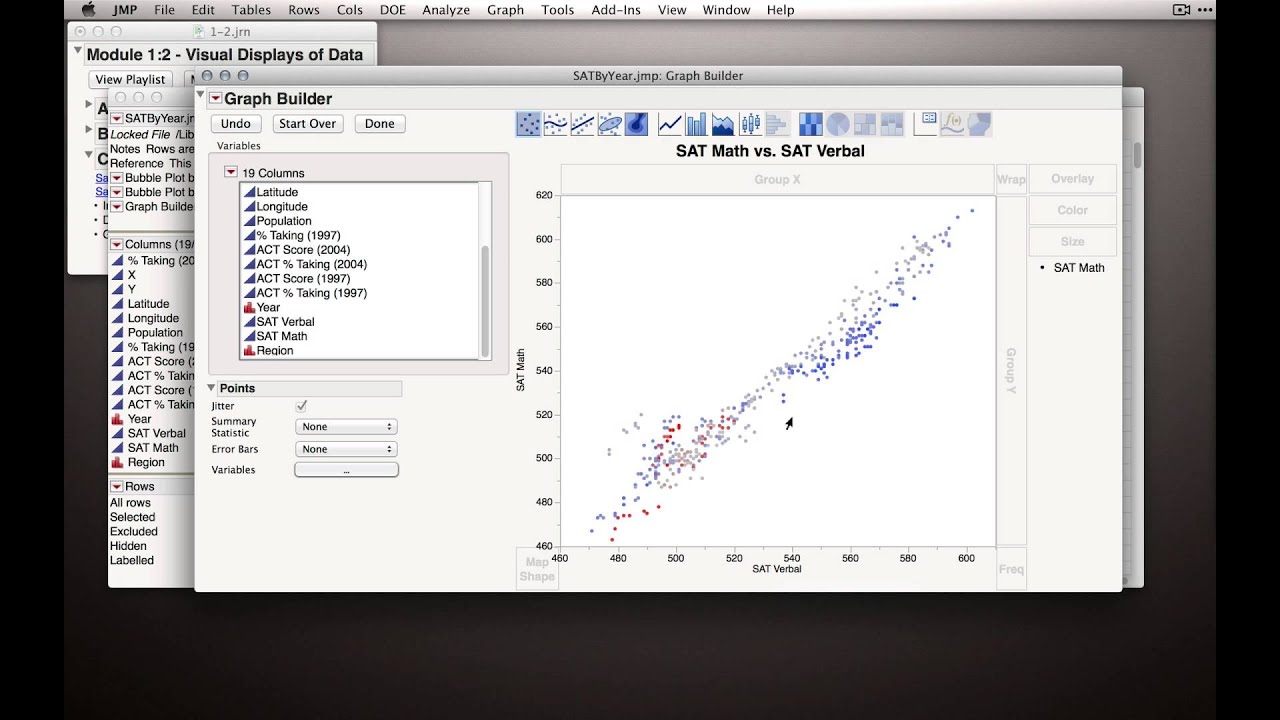
It’s amazing how many big data strategies place the emphasis on the data technology rather than on the data itself – but data is truly at the center.
